天气预报模式
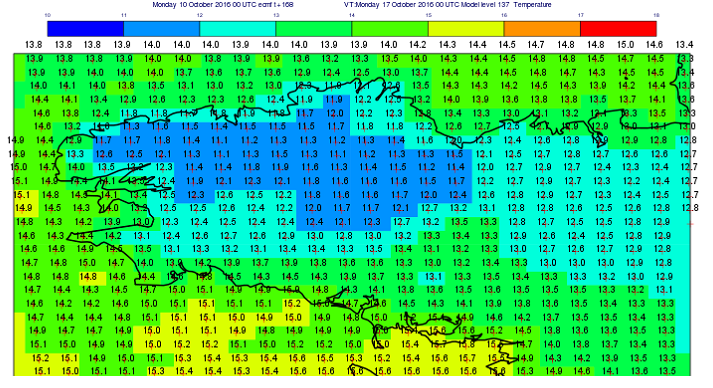
本文介绍了数值大气模拟的基本原理。首先,本文解释了如何将流体力学的一般和连续方程转变为这些方程的离散化形式,随后展示了如何将方程转化为高性能的计算机程序,即大气模式。大气模式可以通过气象观测数据构建的初始状态计算出大气的未来状态,因此,大气模式是数值天气预报系统的核心。
1. 什么是大气模式?
大气模式是模拟大气行为的计算机系统。是一个可以用来研究并更好理解大气过程的数值试验工具,也可用来辅助预测天气和气候。
流体力学定律是描述大气状态演变的基本定律。它描述了流体(液体或气体)从其假定的初始状态随时间的演变过程。它的一般形式是通用的,因此适用于许多差异很大的场景,例如描述飞机周围的空气流动,管道或河流中水的运动,风力涡轮机周围的声波传播,或者烟雾从烟囱上升的过程。这些方程也使得描述海洋和大气等地球物理流体的演变成为可能,这也是大气模式领域的热点问题。研究大气时,流体力学定律被用来预测大气参数,如风、温度、气压和湿度。因为参数之间有很多交叉的相互作用,所以方程都非常复杂。这些方程涉及到的过程包括了从行星的尺度到雨滴的尺度,跨度很大,这些过程与下垫面(陆地、海洋、植被覆盖,见生物圈、水圈和冰冻圈模型)和空间的相互作用也有很大不同。它们的极端复杂性特别是非线性,使方程没有解析解。因此,唯一的解决办法就是用近似的数值技术来计算从初始状态开始的演化。这种被称为分析场的初始状态,本身就是利用高度复杂的数学过程和数值方法来同化大气观测得到的结果(参见气象资料同化)。
大气流体本质上是一种由干空气(20%氧气,80%氮气)和水汽组成的气体,其他气体,如臭氧或二氧化碳,浓度很低(见地球的大气层和气体层)。然而,这些痕量气体在大气的能量平衡中起着至关重要的作用。在大多数天气事件所发生的气层中,对流层、温度和气压条件可能会导致水的相变。因此,水汽可以转化为液体或凝结成冰,形成云和降水。然而,如果要考虑这些相变,在正确地对大气运动建模的过程中,就需要格外关注那些具有非线性阈值因此很难转化为方程的现象。
在数值天气预报(NWP)的背景下(见天气预报介绍),大气模式是业务预报中心实施的“数据同化-计算-预报”循环中的一个环节。它必须能给出高质量的预测,同时还要兼顾快速和稳健,才能按时、准确地提供预报员所需的信息。
2. 流体力学方程
描述了流体力学和热力学基本原理的欧拉方程组是大气模式的基础。这些方程不适用于单个的气体分子,而适用于一个空气团。理想情况下,我们假设空气团既大到包含了足够多的分子来定义温度和压力等统计参数,又小到在广阔的大气环境中可以被同化为一个点。该系统包含三个主要方程:
- 力学方程描述了力施加给气团时,气团运动的加速度和力的函数。在水平方向上,这个方程可以计算出风的加速度,它是压力和地球自转引起的科里奥利惯性力的函数。在垂直方向上,运动的加速度受重力和阿基米德力的控制。
- 总能量守恒方程与由运动方程导出的动能方程相结合,可以得到温度(或由温度导出的另一个量,如焓、熵)的方程。特别是,它描述了太阳辐射、地球或云层发射的红外辐射以及由水的状态变化(蒸发吸热、凝固放热)引发的对温度的影响。
- 质量守恒方程保证了气团的不同成分之间的守恒。
在这三个方程的基础上,补充了气体状态方程和热力学定律,前者在温度、压力和密度三个热力学参数中建立联系,后者描述了水的相变。
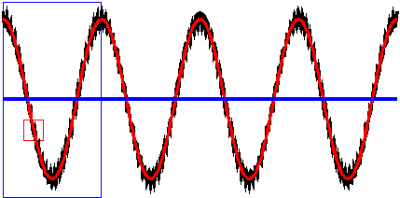
黑色曲线表示的是欧拉方程的解,同时包含了大尺度变化和非常小尺度的波动。蓝色曲线表示较大蓝色方框内的平均解。我们看到,如果我们选择在这么大的窗口内对解进行平均,我们将丢失方程解中包含的所有关于变化的信息。如果我们选择一个较小的窗口进行平均,例如这里的红框内的部分,我们就可以过滤黑色曲线上出现的小的波动,但保留了大尺度的变化趋势(见红色曲线部分)
例如,欧拉方程能够描述地球表面附近的每一个小漩涡,或者地球表面的障碍物周围的气流,但是在实践中,我们用来求解这些方程的计算机的算力还不足以计算出所有这些细节上的演变。因此,简化欧拉方程,只保留气象学的必需项是一项十分必要的工作。简化的第一步是在时间和空间上对原始方程进行平均,以便过滤掉无法用方程描述的快速和小尺度的空间过程。作为这种平均操作的结果,由方程预测的诸如风或温度之类的大气参数不再表示一小块空团的风或温度,而是表示了在一个大到足以包含大量小涡流的空间范围内的平均值,但同时这个空间也不会很大,使得这些平均参数仍然包含了足够多的变化细节,用于描述要预测的天气现象(图 1)。
简化的第二步是分析方程中每个项的量级,以便消除相对于平均后的方程尺度可以忽略的那些项。例如,大气模式中的经典近似是流体静力近似,它忽略了垂直运动方程中的垂直加速度。方程式中的保留的项–重力和阿基米德力–必须相互平衡。因此,在这种构造下,由流体静力学方程(也称为原始方程)包括的平均参数总是处于流体静力平衡状态。换句话说,在这样的模式中,应用于方程的空间和时间上的滤波让实现流体静力平衡的调整过程被视为一个非常快速的过程,不再由方程细致地描述。特别是传播速度极快的声波垂直传播,气象学领域对这一部分并不关注,流体静力学方程也不再考虑声波的垂直传播。对方程进行滤波也是数值模式保持稳定的一个优势(见第3节)。只要大气参数是在水平方向截面大于几公里的范围内的平均值,流体静力近似就是有效的。然而,对于更精细的尺度,显式地描述非静力瞬变过程可能变得很重要。
如果说滤波操作使方程失去了求解大气流体的每一个小细节的可能性,但他保留了表示这些波动对平均场的总体影响的可能性。考虑到与下垫面的交换(生物圈、水圈和冰冻圈模型),这种可能性尤其重要,因为这涉及非常精细和快速的湍流尺度过程。现有的经过实践验证的统计回归规律使得我们能够将地表之上几百米处的小涡旋和微环流的集体效应与这一层的风、温度、湿度的平均演变联系起来。这些统计定律构成了小模式的基础,再把它们集成到全球模式中,称为物理参数化。
水汽会变成水滴或冰晶,随后可以蒸发或者融化,这一系列水的相变不能用平均方程来明确描述。为了能描述它们,有必要采用另一类参数化方案,它基于大量的水滴或冰晶的行为进行统计学描述,被称为云微物理方案。
建模人员还面临着额外的难题,即如何用模式表示因为存在平均垂直速度,而小到不能通过气流上升而产生的云。描述水平范围较小的云(如积云或积雨云)的专门参数化既解决了垂直速度波动问题,也解决了水的相变问题。这些被称为对流方案的参数化是正确表示热带地区能量交换的关键要素之一,从而确保模式可以维持气候的平衡。
建立大气与来自太空的太阳辐射和主要由地球表面发射的红外辐射之间的所有相互作用的模型也是非常有必要的,但这些影响必须经过大量的简化,使辐射的参数化满足业务中心生产预报的限制。
一般而言,大气模式建模人员将方程组中可以直接表示成子系统(称为动力过程)中平均参数的函数的项,与需要在第二个子系统(称为物理过程)中进行参数化过程的项分开,这两个子系统之间的耦合构成了一个大气模式。
3. 离散化算法和数值算法
这些方程不是针对每个网格独立求解的,实际上,描述各种量的在水平和垂直方向上变化的表达式,以及描述这些量随风传输的表达式,都涉及到相邻网格中的值。另一方面,参数化过程也通常涉及使用沿着垂直方向的所有网格进行计算(例如,描述降雨和海洋表面蒸发的水汽通过湍流向上输送的过程)。
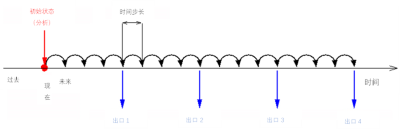
方程的离散化不仅发生在空间上,也发生在时间上。在时间尺度上,时间步长的概念就相当于空间中网格的大小,它将大气模式估算的两个连续的大气状态分离开(图3)。
即使是最复杂的数值方案,也是一种近似求解的方法,当模式的时空分辨率变得非常精细时,其解收敛于精确解。因此,模式的分辨率随着计算机算力的提高而增加。
必须保证在模拟过程中误差水平保持稳定。如果某些条件不满足,某些数值方案的误差将会显而易见地快速增长。一个被称为 CurrentFriedrich-Levy 条件或 CFL 条件[1]的稳定性约束因素,规定一个网格中的风在单位时间步长内携带的空气体积不能超过网格的大小。如果数值方案不满足这一条件,则误差会增加至无穷大,此时方案会变得不稳定,或者说模式就爆炸了。
过去人们已经提出了许多建模方案,而且每年都会有新的方案问世。建模师所面临的难题在于如何在计算时间的精确度、稳定性和效率之间做出最佳的取舍。这些选择和要使用的计算机体系结构也息息相关。
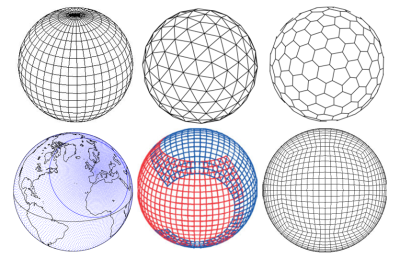
大气模式使用的水平网格类型示例:目前在英国气象局等地使用的规则经纬网网格、德国二十面体非流体静力(ICON)模式中使用的三角形网格、六边形网格、法国气象局大尺度小尺度研究行动(ARPEGE)模式使用的倾斜和拉伸的高斯网格、加拿大使用的阴阳网格、立方体球面网格。
即使所有模式都基于相同的方程组,但在实践中,不同的模式的具体实现可能会有很大的不同。在水平方向上,网格的大小多种多样(图4),包括了由纬线和子午线交叉得到的简单规则网格和具有六边形或三角形格网的网格。一些更奇怪的网格,如立方体球体或阴阳网格,其数学性质十分有趣,特别是它们在两极处并不体现奇点的性质。在某些情况下,网格类型取决于所选用的数值方法。法国气象局的全球模式和欧洲中期气象预报中心(ECMWF)的模式使用了一种数学变换,该变换使用球谐函数来投影参量场。为了使这种变换准确,有必要使用高斯格网[2]。法国气象局也会对格网进行拉伸,以提高法国上空的分辨率。
蓝色的线描绘了模式计算的平均参数所在的位置。这些高程水平线在靠近地表面时随地形起伏,并在海拔较高时趋于水平线。模式用高程水平线上计算的参数表示层中的平均值,即图中红线之间的部分。(见参考文献[3])[来源:Malardel,2009。]
除了为整个大气层提供预报的全球模式,和对于季节或气候等长期变化进行预测的版本外,有许多区域模式只针对给定区域进行计算。在这些区域限定的模式中,计算点数量的减少使得模式得以提高分辨率,并更好地优化该区域的参数化。区域模式的局限性之一是需要将区域边缘上的解与较低分辨率的全球模式提供的解相耦合。区域性传播误差的一个重要来源就是耦合技术,这也限制了这类预测的可持续时间。
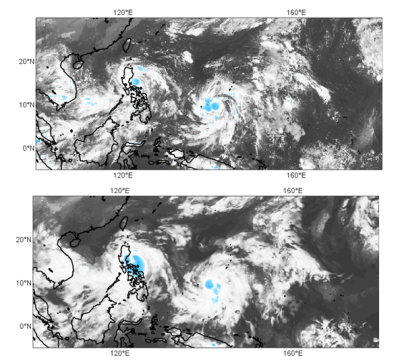
并不是模式在每个时间步长模拟出来的场都会被存储,只有用户所需要的预测时间的数据会被储存。温度、风或重力位势高度等原始参数场被插值到预报员通常使用的等压层。云参数化直接可以提供云量和地面降水信息。从模式的原始输出中确定出的许多其他参数可以帮助预报员分析大气行为,并将预测的数值与实时获取的新观测结果进行比较。因此,对于地表参数需要常规化制图,如地表2米处的温度、地表10米处的风、阵风,以及卫星图像或合成雷达图像(图 6)。模式的输出是专业预报员最主要的信息来源(参见预报员的作用),他们利用这些数据对未来几天的天气的演变情况提供最优预报,并对全球各个地区的预报进行必要的改进。
4. 计算机程序和超级计算机的挑战
2016年运行的预测模式包括数百万个网格,每个网格大约有10个变量,时间步长为几分钟。他们需要解1亿多个复杂的方程式才能产生一小时的预报。只有使用最强大的计算机,以及高效的算法,才能足够快地产生预报,并及时地传送给预报员。
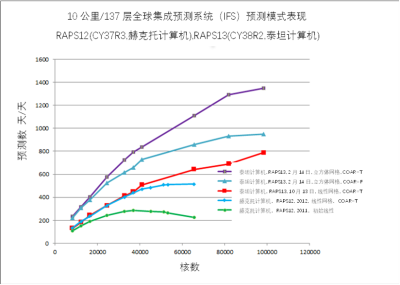
不同的曲线给出了系统可以执行的最大预测天数,具体取决于赫克托和泰坦超级计算机上使用的处理器数量以及不同的系统配置。目前,实际运行上的最低要求是在不到一小时的时间内产生10天的预报,或至少达到每天可以生成240天的天气预报。绿色曲线显示,在2011系统的 Hector ECU 上,系统并行化远非最佳,因为性能没有随着处理器数量的增加而提高(甚至反而降低了)。紫色曲线则显示出,在并行计算方面,系统的性能有了显著的提高。
快速计算的关键之一是能够分散计算,以便使超级计算机的不同处理器并行工作。这种并行化要求将处理器之间的通信降到最少,因为这些通信通常比处理器的本地计算能力慢得多。这些不断演变的技术约束要求模型师不断调整数值格式,以适应超级计算机的新特性。无论是对当前计算机技术的计算速度,还是对未来可能技术的预测速度,都要定期进行比较测试,以评估模式在计算速度方面的效率(图 7)。因此,大气模式其实是由几十个人并行开发和维护的数百万行计算机代码。它是业务化天气预报中心数据同化和高分辨率预报周期的核心。它还被用来产生集合预报中每个成员的扰动预报(见集成预报)。与海洋模式耦合后,它也可用于生产月度和季节性预报,以及监测气候变化(见季节预测)。
大气数值模拟已经并且必须继续解决以下问题:
- 分辨率的增加,因此导致计算次数的增加,
- 要模拟的过程的增加(例如,大气化学过程的增加),
- ECU中处理器数量的增加,
- 超级计算机功耗的增加,
- 程序行数的增加,
- 要处理和输出、存储的数据量的增加。
未来几年内的一个重要挑战是提高现有模式的可扩展性,即它们在适应上述这些所有变化的同时,仍然是可供所有研究人员和开发人员使用的天气预报工具。
参考资料及说明
封面图片:2016年10月10日使用ECMWF数字模式预测的格林威治时间2016年10月17日00时布列塔尼地区的气温(黑色数字:以摄氏度表示的温度;红色十字:网格中心)。
[1] Coiffier, 数值天气预报的元素,《课程与手册》,法国气象局。
[2] Malardel, N. Wedi, W. Deconinck, M. Diamantakis, C. Kuhnlein, G. Mozdzynski, M. Hamrud,Smolarkiewicz : A new grid for the IFS, 2016, ECMWF Newsletter 146.
[3] Malardel, 气象学基本要素,第二版,2009,2009, Cépadues éditions
环境百科全书由环境和能源百科全书协会出版 (www.a3e.fr),该协会与格勒诺布尔阿尔卑斯大学和格勒诺布尔INP有合同关系,并由法国科学院赞助。
引用这篇文章: MALARDEL Sylvie (2024年3月11日), 天气预报模式, 环境百科全书,咨询于 2024年4月26日 [在线ISSN 2555-0950]网址: https://www.encyclopedie-environnement.org/zh/air-zh/weather-forecasting-models/.
环境百科全书中的文章是根据知识共享BY-NC-SA许可条款提供的,该许可授权复制的条件是:引用来源,不作商业使用,共享相同的初始条件,并且在每次重复使用或分发时复制知识共享BY-NC-SA许可声明。